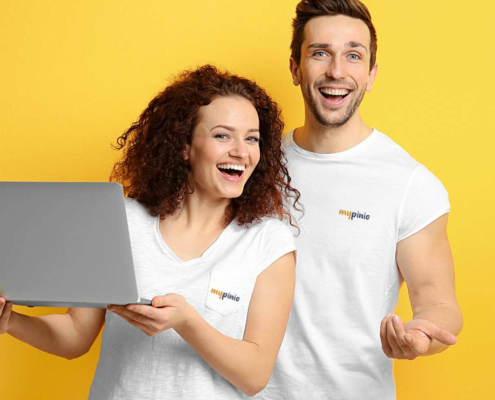
What does Maximum Difference Scaling mean? A definition.
Maximum Difference Scaling, also known as Best-Worst Scaling and often colloquially referred to as MaxDiff Scaling or simply MaxDiff, is a method of market research used to measure consumer preferences, preferences and attitudes for different products, services or other options.
The idea behind Maximum Difference Scaling is that consumers select two objects, one of which represents the best feature or characteristic and the other the worst. The difference between the two selected objects is then taken as a measure of the importance of the feature or characteristic. This process is repeated until all features or properties have been evaluated by all objects.
Maximum Difference Scaling is an effective way to understand and compare customer preferences as it gets participants to choose directly between options rather than selecting a single option.
Maximum difference scaling (MaxDiff) was developed by the American psychologist Jack Knetsch. Knetsch published the first formal description of MaxDiff in his book “The Foundations of Economic Analysis of Law” in 1992. The method has since been widely used in market research and product development and has established itself as one of the most important methods for measuring attribute strengths.
Examples of maximum difference scaling
Example 1:
Suppose we want to find out which of the following leisure activities are the most popular: Walking, Swimming, Cycling and Climbing.
To find out, we can conduct a MaxDiff survey by asking participants to choose between pairs of activities and always select the one they prefer the most. It is crucial that all the properties are in direct comparison with each other.
Please choose which of the following activities you prefer the most:
a) Hiking or swimming
b) Cycling or climbing
c) Walking or cycling
d) Swimming or climbing
e) Climbing or hiking
f) Swimming or cycling
Participants would then select their preferred option and we could analyse the results to determine which activities are most popular.
It is important to note that MaxDiff is not designed to measure people’s absolute preferences, but rather their relative preferences. So it helps us understand which options are most attractive, but it does not give us information about how strong the preferences actually are.
Example 2:
Maximum Difference Scaling is challenging when there are many options, because all options have to be compared. In this case, we proceed as follows:
Let us assume that we do not have 4 leisure activities, but 12, namely hiking, swimming, climbing, cycling, walking, basketball, tennis, long jump, gymnastics, jogging, roller skating and ballet. Since all options would have to be compared with each other, 12 options would result in a total of 66 comparison pairs.This is obviously too many. Therefore, the survey participants are shown only a certain selection from a totality of all items to be evaluated, whereby the respondents must select the most popular and least popular item for them. This is usually done in 3 or 5 rounds. The items are usually shown randomly and may well be repeated.
Session 1:
I like that | I do not like | |
X | Hiking | |
Climbing | ||
Walking | X | |
Jogging |
Session 2:
I like that | I do not like | |
Cycling | ||
Ballet | X | |
X | Basketball | |
Long jump |
Session 3:
I like that | I do not like | |
Tennis | ||
Gymnastics | ||
Roller skating | X | |
X | Swimming |
A meaningful preference model ultimately emerges from the responses of all respondents. The MaxDiff scores are then calculated from the comparison data of the test persons. There are various statistical procedures that can be used to calculate these scores, such as the Thurstone procedure or the Likert procedure. Finally, the scores from Maximum Difference Scaling are interpreted and used to analyse the preferences or attractiveness scores of the objects. The results can be presented in the form of graphs or tables to illustrate the relative strength of the objects’ preferences or attractiveness.
How is the result of the Maximum Difference Scaling calculated?
The result of Maximum Difference Scaling is calculated by determining the relative importance of each attribute compared to the other attributes. There are several statistical techniques that can be used to do this, one of which is the so-called “Normalised Importance Score” method. However, there are other methods that can be used to calculate the results, such as the logit transformation method. It is important to carefully select the method used for the analysis and to keep its limitations and uncertainties in mind.
We outline here two calculation models of Maximum Difference Scaling that you should take a closer look at.
Normalised Importance Score
Normalised importance score is a method used to calculate the relative importance of each attribute in a maximum difference scaling. It works by calculating the number of times an attribute was selected as the “best” attribute minus the number of times it was selected as the “worst” attribute. This gives us a rough idea of the relative importance of one attribute compared to others.
To make the results comparable, the relative importance of the attributes should be normalised by dividing them by the sum of all relative importance. This gives us a percentage value indicating the relative importance of each attribute compared to the other attributes.
The Normalised Importance Scores can then be interpreted to determine the relative importance of each attribute compared to the other attributes. An attribute with a higher percentage score is considered more important than an attribute with a lower percentage score.
Logit transformation
The logit transformation is a method used to analyse the results of maximum difference scaling or pairwise comparison methods. It is based on the logit transformation, also known as a logistic function, which is a function that calculates the relative importance of attributes.
The method assumes that the probability of an attribute being selected as the “best” attribute is a function of the difference between the value of the attribute and the value of the comparison attribute.
The logit model calculates the relative importance of the attributes based on the pairwise comparisons. The relative importance is expressed by a probability that describes how likely the attribute is to be chosen.
The logit transformation can be used to analyse the results of maximum difference scaling or pairwise comparison methods by calculating the relative importance of the attributes and plotting the results on a scale from 0 to 1. An advantage of the method is that the results are easy to interpret, as they express the relative importance of the attributes in the form of a probability.
What is your favourite leisure activity? Vote or write it in the comments!
→ Click here for your vote or comment
What are the advantages of Maximum Difference Scaling?
One advantage of maximum difference scaling is that it provides a simple way to identify consumer preferences by focusing on a small number of attributes rather than assessing many attributes individually. It is also a quick and inexpensive method of collecting data as it requires only a few questions and can be done quickly.
Another advantage of maximum difference scaling is that it has high reliability, as it is based on a clear and easy-to-understand concept and the results are easy to interpret. It is also a valid method as it captures the true preferences of consumers by considering the best and worst features that are important to them.
Overall, maximum difference scaling offers many advantages for collecting data and measuring consumer preferences. It is an effective and reliable method that can be used in many different contexts. Other advantages include:
- Direct comparison: MaxDiff scaling allows participants to choose directly between options instead of selecting only one option. This gives a more accurate picture of participants’ preferences.
- Easy to use: Maximum Difference Scaling is easy to understand and use as participants are only asked to choose between options. It does not require any special training or knowledge.
- Fast results: Max Diff Scaling usually gives quick results as it is a simple method that does not take much time.
- Suitable for large samples: Max Diff scaling is particularly well suited to large samples as it is a quick and easy method that can give accurate results even with large amounts of data.
- Suitable for a wide range of options: MaxDiff is well suited for use with a variety of options as it gets participants to choose directly between options rather than just selecting a single option.
- Suitable for use with other methods: Max Diff Scaling can be used well in combination with other market research methods to gain a comprehensive understanding of participants’ preferences.
- Maximum Difference Scaling is a cost-effective method for collecting data as it requires little time and resources.
What are the disadvantages of Maximum Difference Scaling?
One disadvantage of MaxDiff is that it only measures consumers’ relative preference for different features or attributes, but does not provide information on how much a consumer would actually pay for a particular feature. Because of this limitation, it is difficult to estimate consumers’ actual value preferences.
Another disadvantage of MaxDiff is that it may not capture all the relevant features or attributes that consumers consider when deciding on a particular product or service. If important characteristics are not captured, the results may be inaccurate or biased.
Another potential drawback of MaxDiff is that it may not be well suited to measuring the preferences of consumers who consider a very large number of characteristics or attributes. In such cases, MaxDiff results could potentially be unreliable or inaccurate.
Some other possible drawbacks of Maximum Difference Scaling are summarised as follows:
- It requires a large enough sample: the accuracy of the results depends on how representative the sample is. If the sample is too small, the results may be inaccurate.
- It can be difficult to select the right items: To measure customers’ preferences and attitudes, the items used in Maximum Difference Scaling need to be carefully selected. If the items are not well chosen, the results may be biased.
- There may be bias due to “anchoring” effects: If an item is presented first, this can lead to the scores of the following items being influenced by it. This can lead to distortions in the results.
- There may be problems with scaling: Maximum Difference Scaling uses a particular scaling method based on the assumption that participants’ preferences correspond to a linear scale. However, this assumption may not always be valid, which can lead to bias in the results.
- There may be problems with comprehensibility for participants: Maximum Difference Scaling can be difficult for some participants to understand, especially if they have no experience with this type of measurement. This can lead to them not putting enough effort into scoring the items, which in turn can affect the accuracy of the results.
Conclusion
Maximum Difference Scaling is a method of preference measurement that makes it possible to quickly and easily capture the relative importance of attributes in a survey. It works by asking respondents to select from a variety of attributes the one they consider most important/best and the one they consider least important/worst. This allows the relative importance of the attributes to be recorded and compared.
An advantage of Maximum Difference Scaling is that it is easy to perform and the data is easy to interpret. It does not require special training or knowledge of statistical methods to understand the results.
However, there are also some disadvantages to using Maximum Difference Scaling. One of them is that it is usually a subjective method, as respondents express their own preferences and opinions. It can also lead to the possibility of some attributes being overrated, as respondents are asked to focus on the ‘best’ and ‘worst’ attribute.
In summary, maximum difference scaling is a simple and intuitive method to capture relative importance of attributes, but it has its limitations and it would be advisable to use it in combination with other methods.
read also
#marketresearch #netpromoterscore #semanticdifferential #likertscale #insightscommunity
[shariff]
Keywords of this blog post
maximum difference scaling | max diff scaling | maxdiff | example | definition